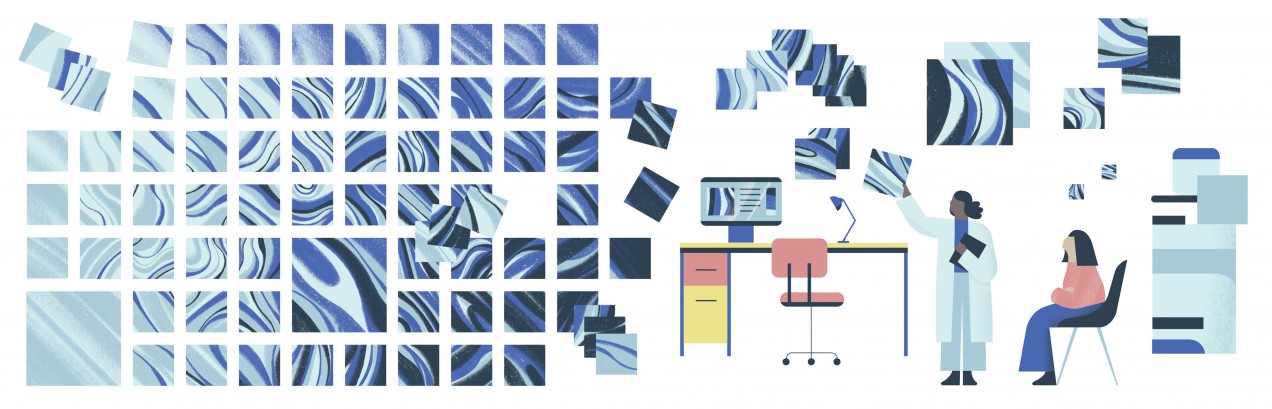
For years artificial intelligence has been seen as the “next big thing” in medicine. Now some MIT professors, students, and alumni are stepping up to make sure it really will be.
Through the Abdul Latif Jameel Clinic for Machine Learning in Health, or J-Clinic, announced last fall, researchers from across MIT will be pursuing projects aimed at developing novel machine-learning methods to improve clinical care, design new medicines, and reduce health-care costs. The initiative will apply AI to a wide range of diseases and builds on ongoing MIT research, including work on drug discovery and early advances in cancer diagnostics by Regina Barzilay, the Delta Electronics Professor in the Department of Electrical Engineering and Computer Science.
Barzilay says it’s time for artificial intelligence to become a standard part of cancer care. “In every single cancer center in the US, be it a community clinic or the top cancer center in the country, there is a serious need to bring AI in,” says Barzilay, a member of both CSAIL and MIT’s Koch Institute for Integrative Cancer Research. After her breast cancer was missed for several years, she began using image-processing algorithms to analyze mammograms. The idea is to go beyond what humans can see in a scan to detect early changes in tissue that mark the path toward cancer.
Institute Professor and Nobel laureate Phillip Sharp, who chairs J-Clinic’s advisory board, says there’s no doubt artificial intelligence and deep learning can—and must—transform medical care. Sharp says that by contributing to earlier diagnoses, AI can improve patients’ quality and length of life. Specifically, he thinks it can transform radiology, make sense of molecular and genetic data to distinguish between malignant and harmless cells, and spot patterns in medical data that can warn of impending problems. He also thinks it can improve the cost-efficiency of medical care by diagnosing disease earlier, when treatment is less expensive and more effective. “We have to get more efficient in health-care delivery,” he says.
Through J-Clinic, he says, MIT will play a crucial role in developing these technologies and training their users, just as MIT has done in molecular biology, cellular biology, genetics, and biotechnology. Barzilay and James Collins, the Termeer Professor of Medical Engineering and Science, serve as faculty co-leads for J-Clinic, a major collaborative effort between MIT and Community Jameel, the social-enterprise organization founded and chaired by Mohammed Abdul Latif Jameel ’78.
AI has taken longer to be applied in health care than most other industries because the stakes are so high. If Amazon tries out a new algorithm that doesn’t work, the company might be out some money. In medicine, people might die. That’s why only 5% of US hospitals reported using some form of artificial intelligence in 2017. But things are finally starting to change. Major hospitals and pharmaceutical companies now invoke AI when they talk about their future. Conferences get broad attendance, and medical AI startups are becoming more common. Computers can now see and read—not as well as people, but they’re getting there, says Michael Hayes, SM ’96, who launched the nonprofit startup CancerAI in 2018 to bring artificial-intelligence tools to market.
Today’s artificial intelligence is based on algorithms that parse gigantic data sets. So-called deep learning, which has advanced substantially in the last decade, allows researchers to draw conclusions from huge amounts of data. Visual and natural-language processing technologies have also improved dramatically. And data storage has gotten substantially cheaper.
“Ten years ago, there weren’t the amount of electronic medical records there are today,” Hayes says. “And even if they did exist, we didn’t have algorithms that could understand doctors’ notes very well and didn’t have computers cheap enough.” The scene now looks very different on all those fronts. “What 10 years ago would have been a supercomputer costing $1 million—that level of computing now can be purchased for a few thousand dollars,” he says. “That has changed the game in a big way.”
Since it was formed last fall, J-Clinic, which is part of the MIT Quest for Intelligence and is chaired by the dean of the School of Engineering, Anantha Chandrakasan, has put out a request for proposals within MIT. So far, professors and students have proposed 43 research projects that would use these advances to benefit patients. Improving diagnosis, targeting treatments to individual patients, and understanding disease progression “are all prediction problems,” Barzilay says. And prediction is where AI excels.
One issue, though, has been that customizing machine-learning algorithms for clinical settings involves training them with what she describes as “massive amounts of manually annotated data.” J-Clinic researchers plan to develop algorithms that aren’t as dependent on hand-labeled data—and that can use data from related domains to fill in gaps in the target area. “Rather than training supervised-learning systems for each individual hospital system and for each disease, we are developing algorithms that can be easily adapted to new settings and different diseases,” Barzilay explains.
Protecting patient privacy and ensuring that the data reflects the diversity of the population are also key goals of J-Clinic. Researchers are developing algorithms that can perform computations on encrypted data, so patients don’t need to fear intimate health information being left in the open. And J-Clinic is building a large, international network spanning everything from rural clinics to major urban academic hospitals to implement and test the algorithms they develop. The hope is that this will make their work much more generalizable than other health-care algorithms published to date, most of which are trained on data from a single hospital.
The work already under way in the lab of Barzilay, a 2017 MacArthur “genius grant” winner and a leader in the AI field, offers a glimpse into the potential that J-Clinic and startups like CancerAI can help unlock. One area of her research involves using machine learning to accelerate drug discovery. That work helps developers zero in on molecules with promising properties for fighting cancer and a wide range of other diseases. (See “AI is reinventing the way we invent,” MIT Technology Review, March/April 2019.) On the cancer diagnostics front, she’s also one of the first AI researchers to develop a tool that actually helps people.
In a paper published last year in Radiology, she and her colleagues, including researchers from Massachusetts General Hospital, used AI to develop a method for assessing the density of breast tissue. Today, mammograms miss about 15% of breast tumors—and they miss more than half, according to several studies, if the breast tissue is dense, which makes tumors harder to see. More than 40% of American women have dense breast tissue, which also puts them at higher risk for breast cancer.
Barzilay and her colleagues used more than 41,000 digital mammograms, evaluated and classified by experts, to train a deep-learning algorithm to assess density so that women who may require extra screening can be identified. In a six-month trial looking at over 10,000 mammograms, the model agreed with Mass. General radiologists 94% of the time, making it the first time this kind of deep learning had been used successfully in a clinical setting. Barzilay and her collaborators now hope to scale their system up to other hospitals.
Barzilay is also using AI to detect the earliest changes on the road to breast cancer—changes that a pathologist can’t see. “Cancer doesn’t grow from today to tomorrow. It’s actually a very long process, which makes a lot of changes in tissue,” she told the audience at the “Hello World, Hello MIT” conference celebrating the launch of the MIT Schwarzman College of Computing in February. She showed two mammograms, one from a woman who had gone on to get breast cancer two years after the scan. “The logical question is: can you take the machine and train it on the images, when we know the outcome in two years or five years, to say what is there to come?” As it turns out, she said, “the machine was able to do this task pretty well.” Barzilay, her grad student Adam Yala ’16, MEng ’17, and Constance Lehman, head of breast imaging at Mass. General, developed a model that identified characteristics often preceding the appearance of cancer—and if those characteristics show up in a mammogram, the patient can be flagged.
In late February, physicians at Mass. General began testing that risk model. A woman whose mammogram places her in the riskiest 20%, Barzilay says, has “a very nontrivial chance to get breast cancer.” Now, doctors at Mass. General are working to figure out how to use that information to change her odds.
That vision for artificial intelligence is a far cry from the current use of digital technology in doctor’s offices, which is mostly limited to electronic medical records that have yet to live up to their potential. Such systems can leave doctors burned out, forcing them to devote such long hours to inputting data that they spend more time with their computer screens than their patients.
But on the plus side, electronic records have allowed hospitals to amass huge quantities of patient data that AI researchers hope will eventually pay dividends for patients, caregivers, hospitals, and insurers.
As J-Clinic and startups like CancerAI begin tapping into that data, Collins, J-Clinic’s other faculty co-lead, says he sees J-Clinic not just bringing together AI experts, medical experts, and data sets to advance medical research but also helping translate that research into the clinical setting. It’ll do this, he says, by getting early technologies into hospitals for testing and validation, and by facilitating the launch of companies to commercialize them. He also envisions J-Clinic initiating a public discussion around what he calls the “promise and perils of AI and health care”—and asking hard questions about how to enhance existing care, reduce costs, protect patient privacy, and ethically obtain useful data.
Although technological innovation usually drives up medical costs, he hopes that artificial intelligence will be an exception, perhaps by maximizing bed usage, limiting the time doctors spend on administrative duties, and developing drugs more economically. “I’m curious as to the ways AI can help with efficiencies in health care—whether it’s bed usage, scheduling, billings—to squeeze out the administrative overhead there,” says Collins, whose wife is a physician. He thinks that the administrative burden of electronic medical records could be reversed with better technology, potentially leading to savings.
In his own lab, Collins, a synthetic biologist, plans to use AI platforms to better identify novel classes of antibiotics and cancer drugs, among others. “I’m keen to explore in what way AI can be used more broadly as a useful assistant in the context of research and potentially in the context of medicine,” he says.